You are here:
- Home »
- Using evidence for learning »
- Gathering evidence »
- Data analysis
The principles of data analysis
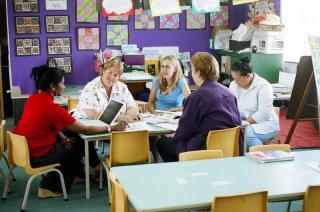
Although collecting accurate assessment data is a challenge for schools, a bigger challenge is analysing the information effectively and acting on it to effect change in the classroom.
A good data analysis process comprises several important steps. While it is not necessary to be overly rigid or formal about this, it is advisable to give some attention to following these steps, especially for people who are new to the analysis process.
Step 1: Begin with some clear, high-level questions. What is it that you want to know? A strong data analysis should always have one or more objectives that will form the basis for high-level questions. It is important to make the questions as clear, specific and well-defined as possible. "High level” means that the questions need not be framed in terms of the data. When the data are considered it might turn out that some of the high-level questions cannot be addressed, or addressed only imperfectly. In this sense, the high-level questions form a sort of a knowledge wish list.
Step 2: Consider the available data and consider which data can be used to inform the high-level questions.
Step 3: Design lower-level questions that are framed in terms of the available data. For example, a high-level question might be, "How do boys and girls compare in terms of progress in mathematics during the primary school years?".
- The available data might be a series of scores from a standardised test completed by each child in each year.
- The lower-level questions might be: "What are the mean test scores for boys and girls in each year level? "What are the mean differences?" and "How do these differences change over successive years?"
Step 4: Design actual analyses, carry them out, and interpret their results.
- Using the example of comparing boys’ and girls’ progress in mathematics, the analysis might involve calculating each student’s quantitative scale score in each year level from the raw test data, and then investigating whether there is an interaction between gender and time.
- When the analysis has been carried out, the original, high-level questions should be revisited in light of the results. For example, we might find that overall, primary school boys are, on average, ahead of girls in mathematics at all year levels, that the average level of performance of both boys and girls tends to improve each year (that is, they make progress), and that there is an interaction between time and gender such that the difference in performance between girls and boys tends to peak in about year 4 and thereafter diminish over time (that is, that girls begin to catch up). From this, we might conclude that boys tend to make more initial progress than girls, that girls make more progress subsequently, but not enough to perform at the same level, on average, as boys by the end of primary school.
Step 5: Make explicit limitations of the data and analysis, especially when it is to be reported to other people. In this case, an important limitation would be that mathematics performance was measured using a single test. This means that any important aspects of mathematics not assessed in that test will obviously not be represented in the analysis. Furthermore, there may be test artefacts that limit the validity of the analysis. Other limitations might arise from a small sample size, or a bias in the sample (for example, all students being from high decile schools).
Finally, a good analysis will usually raise new questions. Thus, analysis of assessment data can be seen as an ongoing cycle of inquiry, serving continual improvement in teaching and learning.
For more information, see the next sections: